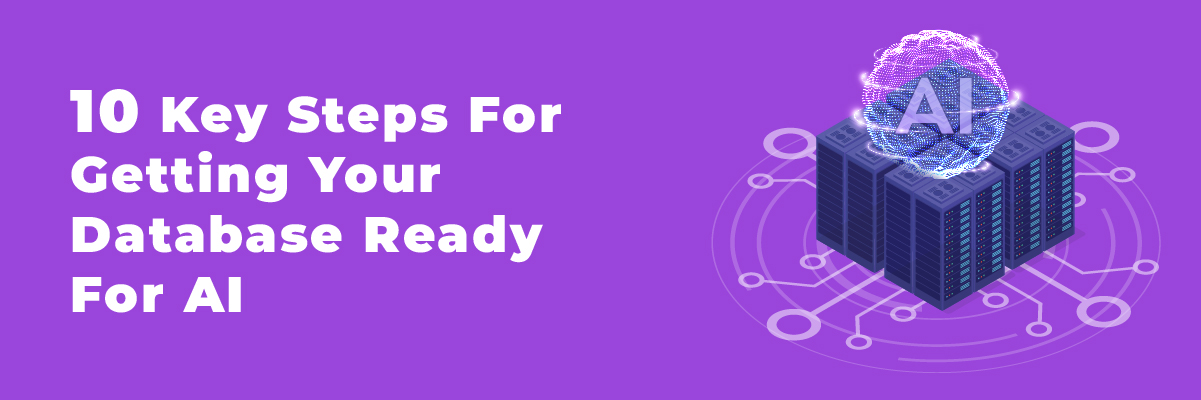
AI is only as good as the data it learns from. Without proper data preparation for AI, organizations risk inaccurate models and unreliable insights. From data cleaning for AI data management models to optimizing big data for AI, ensuring your database is AI-ready is crucial for success.
In this guide, we’ll walk you through 10 key steps to structure, clean, and optimize your database—laying the foundation for seamless AI integration and smarter decision-making. Let’s get started!
We have found that companies increase their chances for successful integration of AI exponentially by following these 10 steps. Please note that these steps are general, and any specific applications need to be discussed thoroughly. If you need help, let us know. We’d be happy to share our experience.
- Data Inventory and Assessment: Conduct a comprehensive inventory of all data sources, including databases, files, and data warehouses. Assess the quality, completeness, and consistency of the data in each source.
- Data Integration and Standardization: Integrate data from different sources to create a unified view of the organization’s data landscape. Standardize data formats, naming conventions, and data dictionaries to ensure consistency and compatibility across datasets.
- Data Cleaning and Preprocessing: Cleanse and preprocess the data to remove inconsistencies, errors, duplicates, and missing values. This ensures that the data is accurate, reliable, and suitable for analysis.
- Data Security and Compliance: Does all data need to be imported into AI, should it all be imported? Implement robust data security measures to protect sensitive information and ensure compliance with relevant regulations such as GDPR, HIPAA, or industry-specific standards. Establish cloud databases for AI, access controls, and encryption mechanisms to safeguard data privacy and integrity.
- Data Governance Framework: Establish a data governance framework to define policies, procedures, and responsibilities for managing and governing data assets. This includes data stewardship, metadata management, and data lineage tracking to ensure accountability and transparency.
- Data Storage and Infrastructure: Evaluate the scalability, performance, and cost-effectiveness of existing data storage and infrastructure solutions. Consider migrating to cloud-based platforms or implementing data lakes to accommodate growing volumes of data and enable flexible analytics capabilities.
- AI Readiness Assessment: Assess the organization’s readiness and maturity level for implementing AI data management solutions. Evaluate factors such as data readiness, technological capabilities, organizational culture, and leadership support.
- Skills and Training: Invest in training and upskilling employees to develop the necessary skills and expertise in data science, machine learning, and AI technologies. Encourage a culture of continuous learning and experimentation to foster innovation and adoption of AI-driven insights.
- Pilot Projects and Proof of Concepts: Test first with smaller datasets. Start with small-scale pilot projects or proof of concepts to demonstrate the value and feasibility of AI applications. Identify specific use cases or business problems where AI can provide tangible benefits and measurable outcomes.
- Collaboration with AI Experts: Collaborate with AI experts, data scientists, and technology partners to leverage their domain knowledge and technical expertise in implementing AI solutions. Consider outsourcing certain aspects of AI development or consulting services to accelerate the implementation process.
The Role of Data Quality for AI
The significance of data quality for AI cannot be overstated. Data serves as the foundation for every data preparation for AI initiative, dictating the accuracy and effectiveness of its decisions and predictions. It’s not merely about quantity; quality plays a pivotal role in shaping intelligence.
Data cleaning for AI models must undergo meticulous training with a keen focus on data quality, akin to ensuring the clarity of a lens for accurate perception. Distorted or clouded data compromises the AI’s ability to comprehend and respond effectively.
When addressing data quality, precision, reliability, and relevance are paramount. Similar to how a dependable compass guides a traveler, high-quality data directs AI models. Implementing Big Data for AI for data quality involves employing robust Data Cleaning Techniques to ensure accuracy and reliability. Successful AI implementation hinges on Ensuring data quality, enhancing AI accuracy, and ultimately optimizing outcomes.
Steps of preparing a solid data foundation for AI
To ensure successful generative AI or cloud databases for AI, implementation and drive positive business outcomes, follow these strategic tips:
- Define Clear Goals: Identify your project goals and specific business challenges or opportunities before diving into generative AI. Clear goals help create an effective implementation roadmap.
- Curate Diverse Data: Gather a diverse dataset relevant to your business objectives to enable the generative AI model to comprehend and generate outputs that reflect real-world complexity. For example, an e-commerce platform should collect diverse data like customer purchase history, browsing behavior, and demographics to provide personalized recommendations.
- Prioritize Data Quality: Focus on data quality over quantity. Use tools for data profiling, cleansing, validation, and monitoring to eliminate inaccuracies and biases. A healthcare software provider, for example, should ensure patient records are accurate to enhance AI diagnostic insights.
- Integrate Data Sources: Create a unified view by integrating data from various sources and formats. This improves accessibility and minimizes inconsistencies. An ERP software provider can integrate data from different departments to enrich AI analysis across financial, inventory, and customer management systems.
- Label Your Data: Add annotations or tags to make data understandable for AI algorithms. Techniques like data annotation, classification, and verification are crucial. For instance, labeling customer data with tags like purchasing behavior helps AI-driven marketing tools create effective campaigns.
- Augment Your Data: Enhance data quantity, diversity, and quality by creating new or modified data from existing sources. A financial institution can use synthetic data points to improve AI fraud detection models.
- Secure Your Data: Implement stringent security measures, including encryption, access controls, and regular audits, to safeguard sensitive information. A technology company can protect customer data and ensure compliance with privacy regulations.
- Establish Data Governance: Develop policies and processes to manage data throughout its lifecycle. This aligns data quality, integration, labeling, and privacy with AI objectives. An insurance company should have governance policies to manage customer data effectively.
- Regularly Update Your Dataset: Keep your data current to reflect evolving business needs and trends. A finance software provider should regularly update market data to keep AI-driven investment tools relevant.
- Handle Missing Data: Use strategies like statistical replacement or deletion of incomplete records to maintain dataset reliability. A telecommunications company can ensure customer data completeness for accurate predictive analytics.
Unleash the Power of Speed, Stability, and Safety
As artificial intelligence continues to reshape industries, having an AI-ready database is no longer optional—it’s a necessity. Preparing your database for AI involves thoughtful planning, from optimizing data structures to ensuring robust AI database optimization techniques are in place. Leveraging advanced tools and practices, such as scalable AI data center infrastructure, can further enhance your system’s ability to process and analyze vast amounts of data effectively.
When considering how to prepare your database for AI applications, focus on data quality, storage efficiency, and real-time analytics capabilities. A database designed with AI in mind not only boosts performance but also unlocks new opportunities for innovation, enabling your organization to stay competitive in a data-driven world.
Embracing AI starts with the right foundation. Optimize today to meet tomorrow’s challenges seamlessly!
Take the first step towards unlocking the full potential of AI for your business. Contact us today and let’s discuss how our data-first approach and experience can make AI not just a possibility, but a powerful asset for your organization.